Data Preparation
Data Analysis
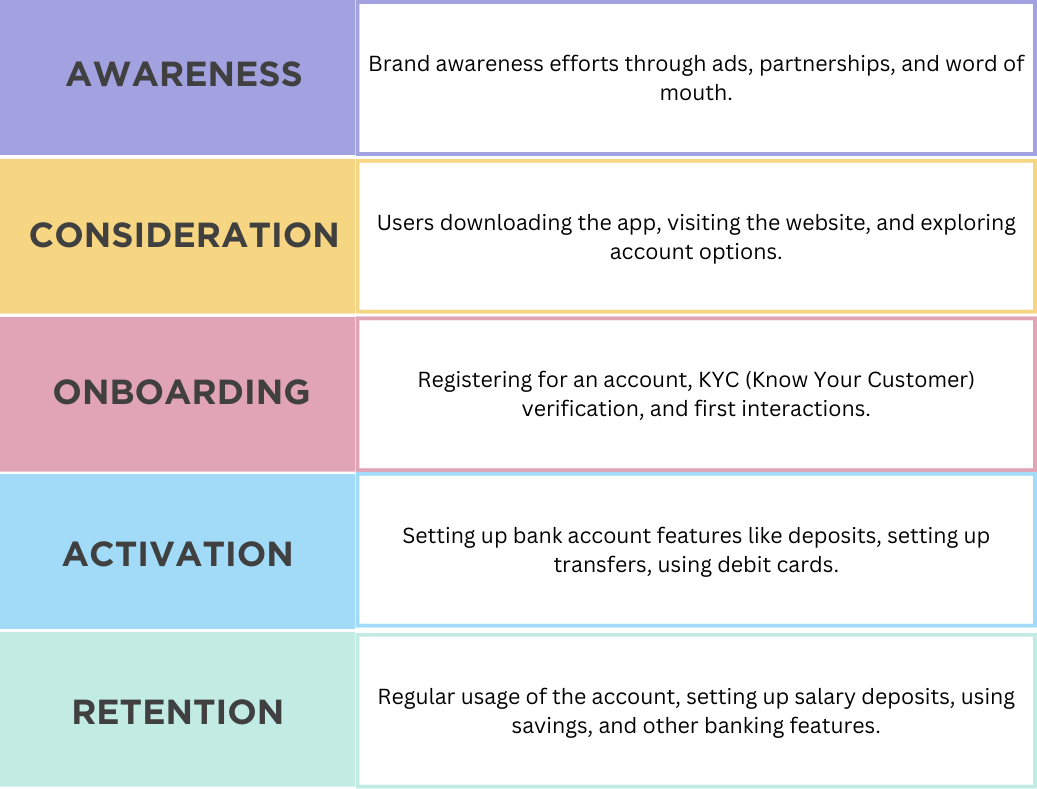
General questions: - How do different countries perform compared to each other? - How do seasonality or monthly trends affect custor's lifecycle stages? - How do external factors (COVID, Laws, BREXIT, Wars, Imigration, change of govermental policies) affect N26 results? - How do internal factors (Market expansion/contraction, new media channels, change in strategy) affect N26 results?
Awareness
To effectively measure awareness, it’s essential to analyze the reach versus interaction levels across different marketing campaigns. This assessment provides insights into how widely the content is seen (reach) and the extent to which it drives user actions (interaction). The main metric for this is Click-Through Rate (CTR), that eveals the percentage of users who engage with the content after viewing it. The dataset provided does not contain enough information to enable the CTR calculation.
Consideration
Conversions:
Onboarding
Activation
User Retention (Cohort Analysis)
Spend
Cost per Acquisition (CPA) - Average cost to acquire an active user Calculations: • Acquisition spend / Net MAU • Compare CPA by country and analyze trends over time Importance: CPA indicates the efficiency of user acquisition efforts, helping optimize spending. Lower CPA suggests a more cost-effective growth strategy and indicates higher return on marketing investments.
General questions: - What is the average unique open rate for the campaigns? Where is there opportunity for improvement? - With only the data provided, which campaign would you say had the strongest body creative/copy? Why? - What’s an audience that you would prioritise for optimisation? Why? Outline an experiment you’d propose to improve performance. - If you could add any column to the dataset, which one would you add and why? ------------------ - How do different countries perform compared to each other? - How do different segments react to the campaigns? Do they behave similarly?
Unique Open Rates - Initial Engagement Calculation: • Moving Average of (unique open rate/emails sent) calculated monthly per campaign - emails don't have a time series that can be used Questions: • Are there any specific email types or topics that consistently stand out in open rates? • Which customer segments show higher open rates, and why? • What improvements could boost open rates (e.g., subject line optimization, send time)? Importance: Unique open rates provide insight into the initial effectiveness of the email’s appeal, indicating how well the subject line, timing, and targeting resonate with recipients. A higher open rate reflects successful targeting and email relevance.
The campaign "Email 12" targeted at the "MAU" segment had the strongest body creative/copy, with an exceptionally high open rate of approximately 604.58%. This suggests that the content was highly engaging or relevant to the audience.
Campaign Engagement - Click Rate Analysis for Campaign Effectiveness Calculation: • Running average of click-through rates, calculated per campaign. Questions: • Which segments show the highest or lowest engagement in terms of clicks? • Do higher click-through rates correlate with lower unsubscribe rates, indicating more effective targeting and content relevance? Importance: Click-through rates offer deeper insight into how engaging and relevant the content is after the email is opened. High engagement suggests that the message aligns with the audience’s interests, while low engagement may signal a need for content adjustments.
Segment Engagement - Evaluating Engagement Across User Segments Calculation: • Running average of click rates by segment, calculated as per segment. Questions: • Which segments are most and least responsive to the content? • Is there a relationship between high engagement rates and lower unsubscribe rates, suggesting better alignment with user expectations? Importance: Segment-specific engagement analysis reveals which groups are most receptive to the content, guiding more personalized and effective messaging strategies for each user group.
The "Never Active" segment shows a relatively low open rate and click rate compared to the "MAU" segment, indicating potential for optimization. To improve performance for the "Never Active" segment, an experiment could be designed to test different subject lines and email content tailored to re-engage this audience. This could involve A/B testing various messaging strategies to identify which approach resonates best with this segment.
The analysis shows that "Email 12" had the highest open rate, click rate, and unsubscribe rate, indicating strong engagement but also a higher likelihood of unsubscribes. Adding a column for "device_type" (e.g., mobile, desktop) could provide insights into how users engage with campaigns across different devices, helping to tailor content for better performance. In terms of country and segment performance, the data does not directly provide country-specific insights, but segment analysis shows that the "Never Active" segment has room for improvement.