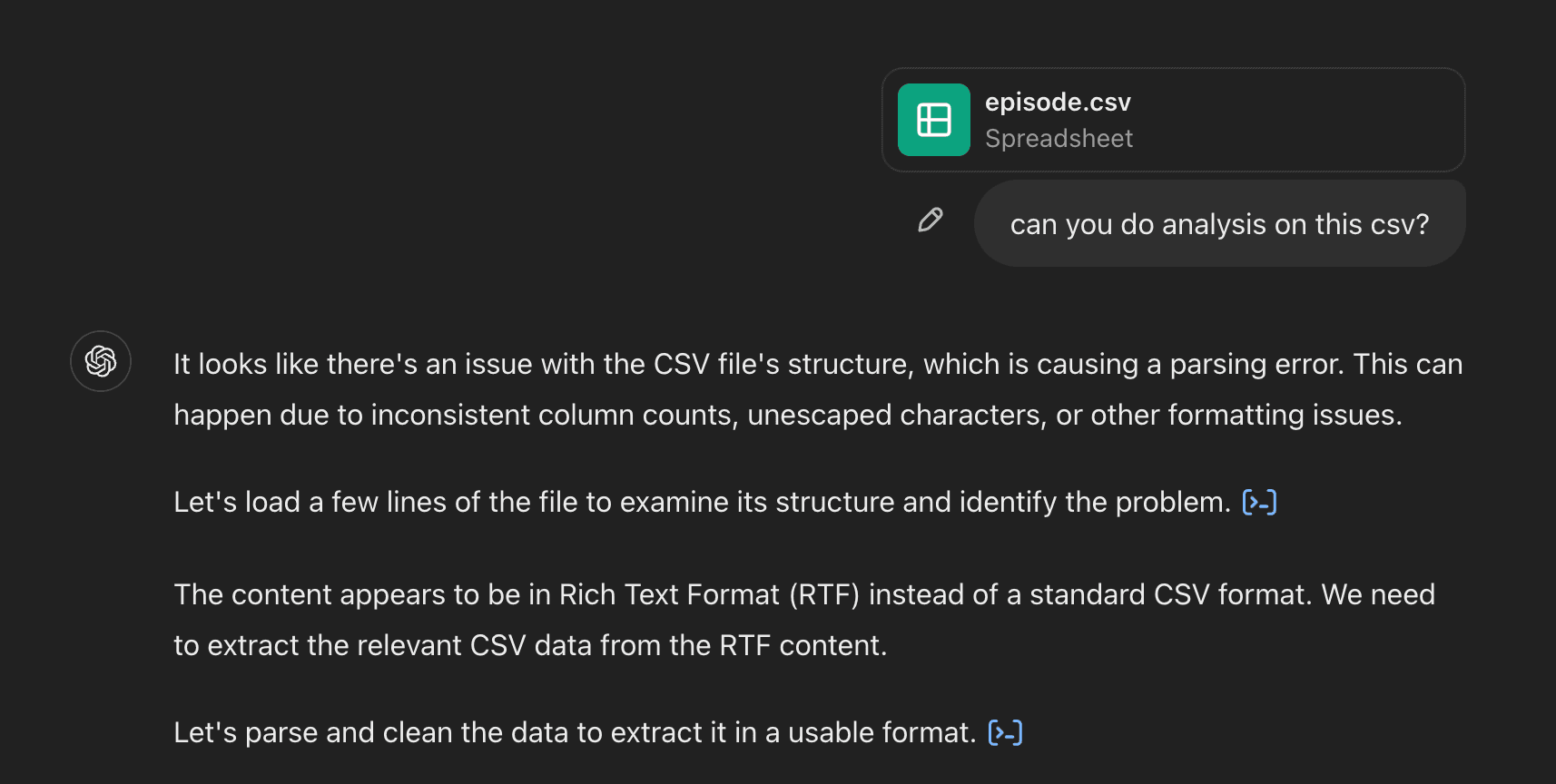
ChatGPT data analysis vs Deepnote:
a side-by-side comparison for 2025
Comparing two data science notebooks.
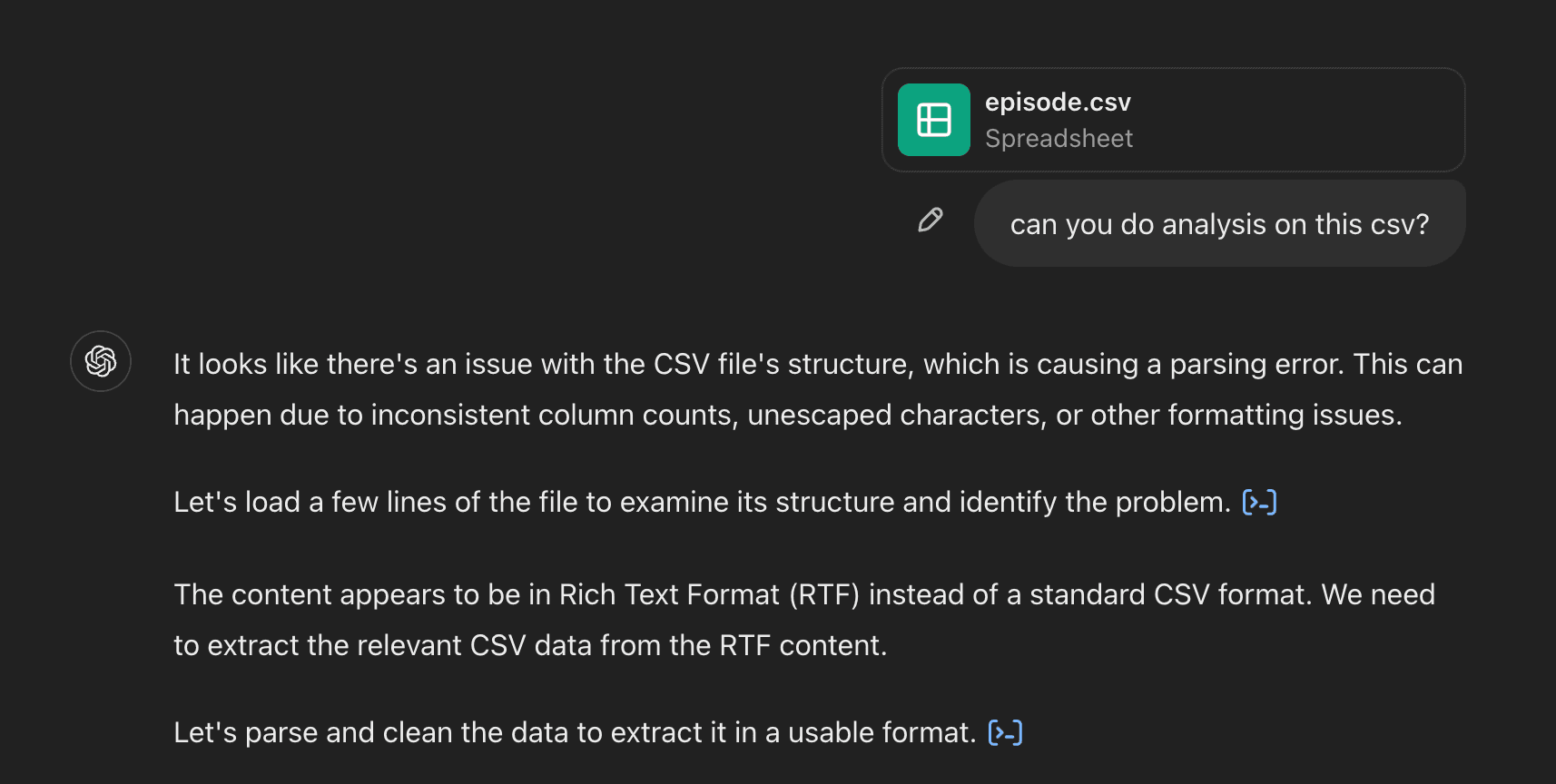
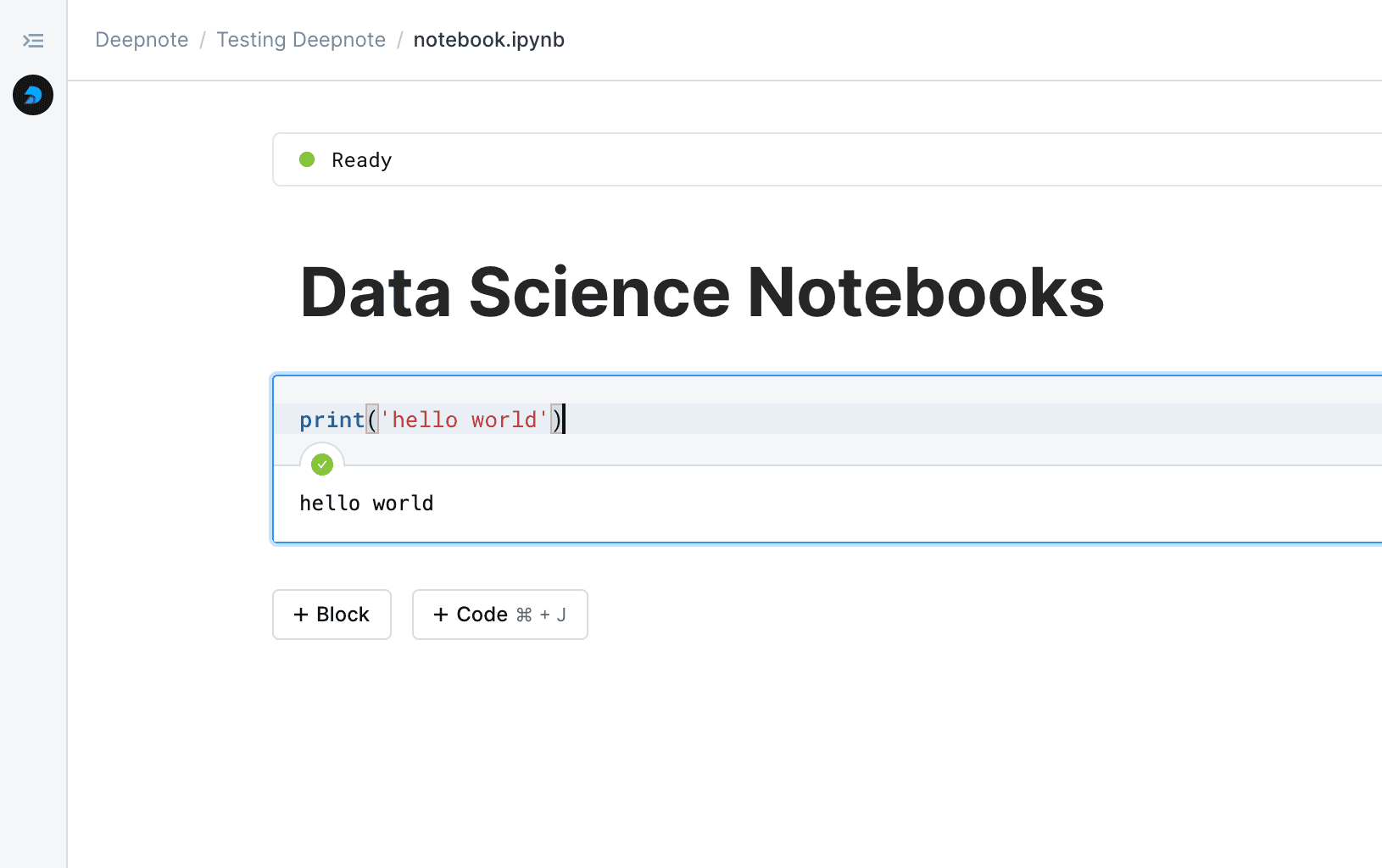
Deepnote
ChatGPT data analysis vs Deepnote
The integration of artificial intelligence into data analysis has created new possibilities for how we work with data. ChatGPT and Deepnote Auto AI represent two distinct approaches to this evolution, each offering unique advantages for different types of users and workflows. Understanding their differences helps in choosing the right tool for specific analytical needs.
Core philosophies
ChatGPT approaches data analysis as a conversational partner, offering guidance and suggestions through natural language interaction. It serves as an intelligent assistant, helping users understand analytical concepts and generate code solutions, but maintains a distance from actual data manipulation. This approach emphasizes accessibility and learning, making complex analytical concepts more approachable for users at all skill levels.
Deepnote Auto AI takes a more integrated approach, embedding AI assistance directly within a comprehensive data science platform. Rather than just suggesting solutions, it provides immediate execution capabilities alongside AI guidance, creating a seamless workflow between ideation and implementation. This integration allows for immediate verification and iteration of analytical approaches.
The analysis experience
Working with ChatGPT for data analysis feels much like consulting with an experienced colleague. Users describe their analytical needs, receive suggestions and explanations, and then implement these ideas in their preferred environment. This separation between consultation and implementation offers flexibility but requires additional effort to put suggestions into practice.
The Deepnote Auto AI experience feels more cohesive. Users work directly with their data while receiving AI assistance, creating a fluid workflow where suggestions can be immediately tested and refined. The platform's context awareness means recommendations are tailored to the actual data and previous analyses, making the assistance more precise and relevant.
Practical applications
The practical application of these tools reveals their distinct strengths. ChatGPT excels in educational scenarios and early-stage analysis planning. Its ability to explain concepts, suggest approaches, and troubleshoot code makes it invaluable for learning and exploration. The platform helps users understand the "why" behind analytical choices, fostering deeper understanding of data science concepts.
Deepnote Auto AI shines in professional settings where immediate implementation and team collaboration are crucial. Its integrated approach allows analysts to move quickly from idea to execution, with AI assistance guiding each step of the process. The platform's ability to work directly with data while providing AI insights creates a powerful environment for professional data science work.
Development and workflow
The workflow with ChatGPT typically involves a back-and-forth conversation about analytical approaches. Users describe their needs, receive suggestions, implement these in their chosen environment, and return for refinement. This iterative process works well for learning and exploration but can become cumbersome for complex analyses requiring multiple iterations.
Deepnote's workflow feels more streamlined, with AI assistance integrated directly into the development process. Users can load their data, receive contextual suggestions, and implement solutions immediately, all within the same environment. This integration reduces the friction between ideation and implementation, accelerating the analytical process.
Collaboration and team dynamics
The collaborative aspects of these platforms reflect their different approaches. ChatGPT's collaboration happens primarily through shared conversations and code snippets, requiring teams to establish their own methods for implementing and sharing actual analyses. This can work well for small teams or individual learning but becomes challenging in larger professional settings.
Deepnote provides a more comprehensive collaborative environment, where teams can work together in real-time while receiving AI assistance. The platform's shared workspaces, version control, and secure data handling create a professional environment suitable for team-based data science work.
Making the choice
Choosing between ChatGPT and Deepnote Auto AI depends largely on your specific needs and context. For individuals learning data analysis or teams exploring new analytical approaches, ChatGPT's conversational interface and broad knowledge base provide valuable guidance and learning opportunities. Its flexibility and accessibility make it an excellent tool for education and initial exploration.
For professional teams requiring a complete data science solution, Deepnote Auto AI offers a more comprehensive approach. Its integration of AI assistance with actual data manipulation and team collaboration features makes it particularly valuable for organizations building data-driven solutions. The platform's ability to combine AI guidance with immediate implementation creates an efficient environment for professional data work.
Conclusion
The choice between ChatGPT and Deepnote Auto AI reflects a broader decision about how you want to approach data analysis. ChatGPT serves as an intelligent consultant, ideal for learning and exploration, while Deepnote Auto AI provides a comprehensive platform for professional data science work.
Many users may find value in combining both tools: using ChatGPT for initial learning and ideation, then moving to Deepnote Auto AI for implementation and team-based analysis. This combination leverages the strengths of both platforms while addressing their individual limitations, creating a powerful toolkit for modern data analysis.