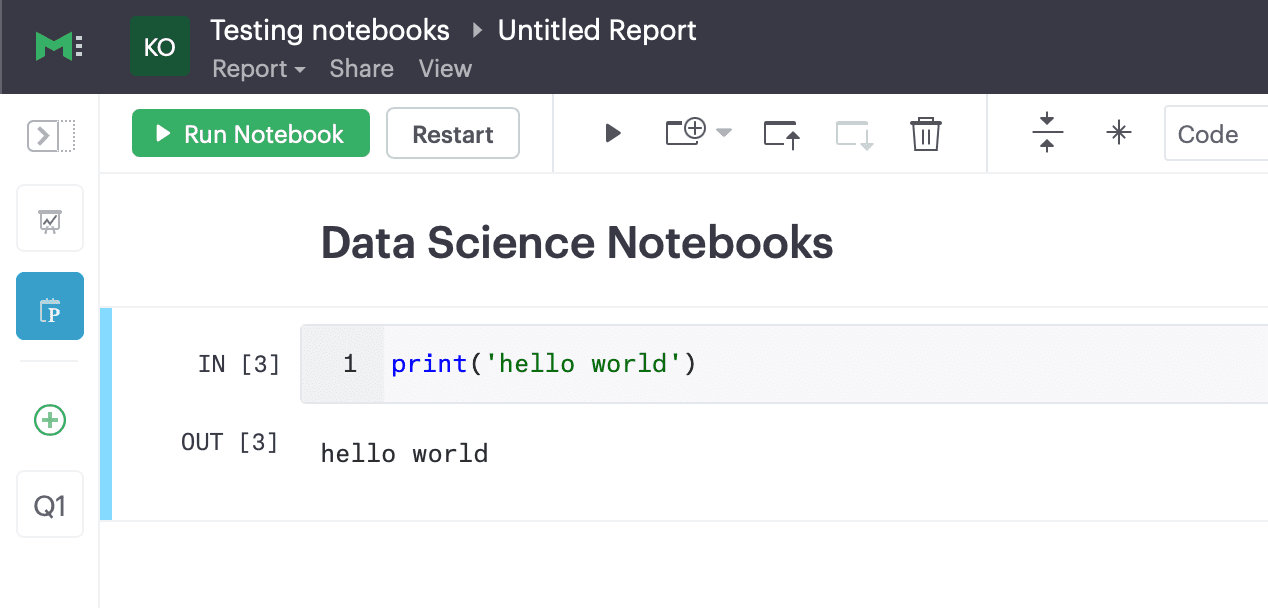
Mode Notebooks vs Deepnote:
a side-by-side comparison for 2025
Comparing two data science notebooks.
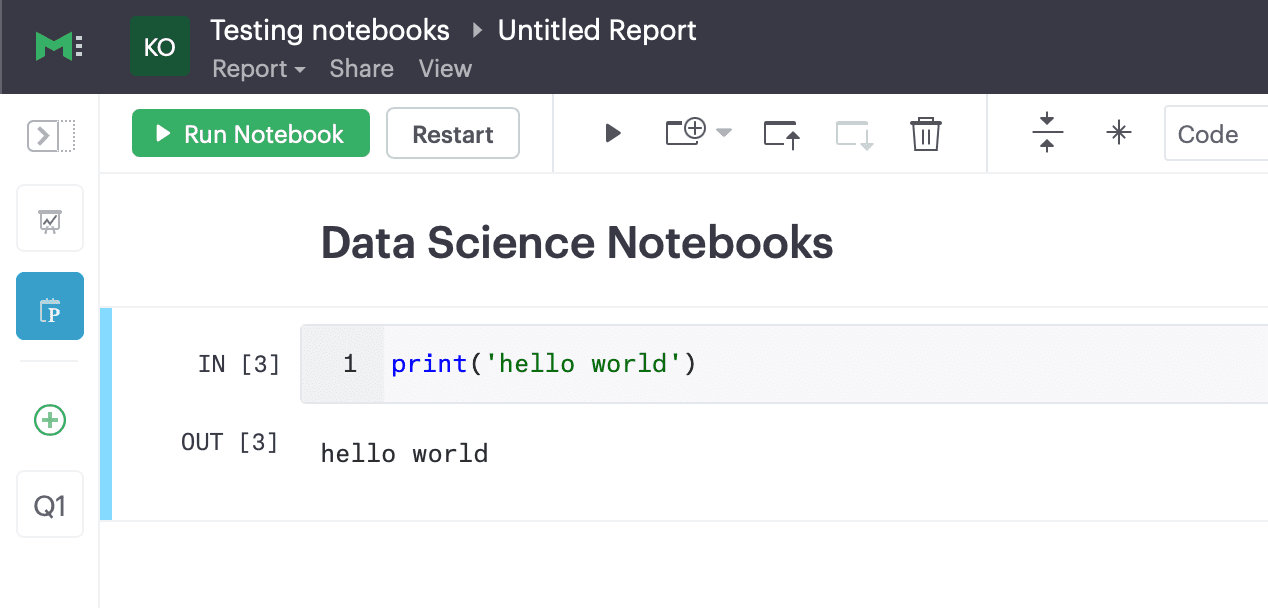
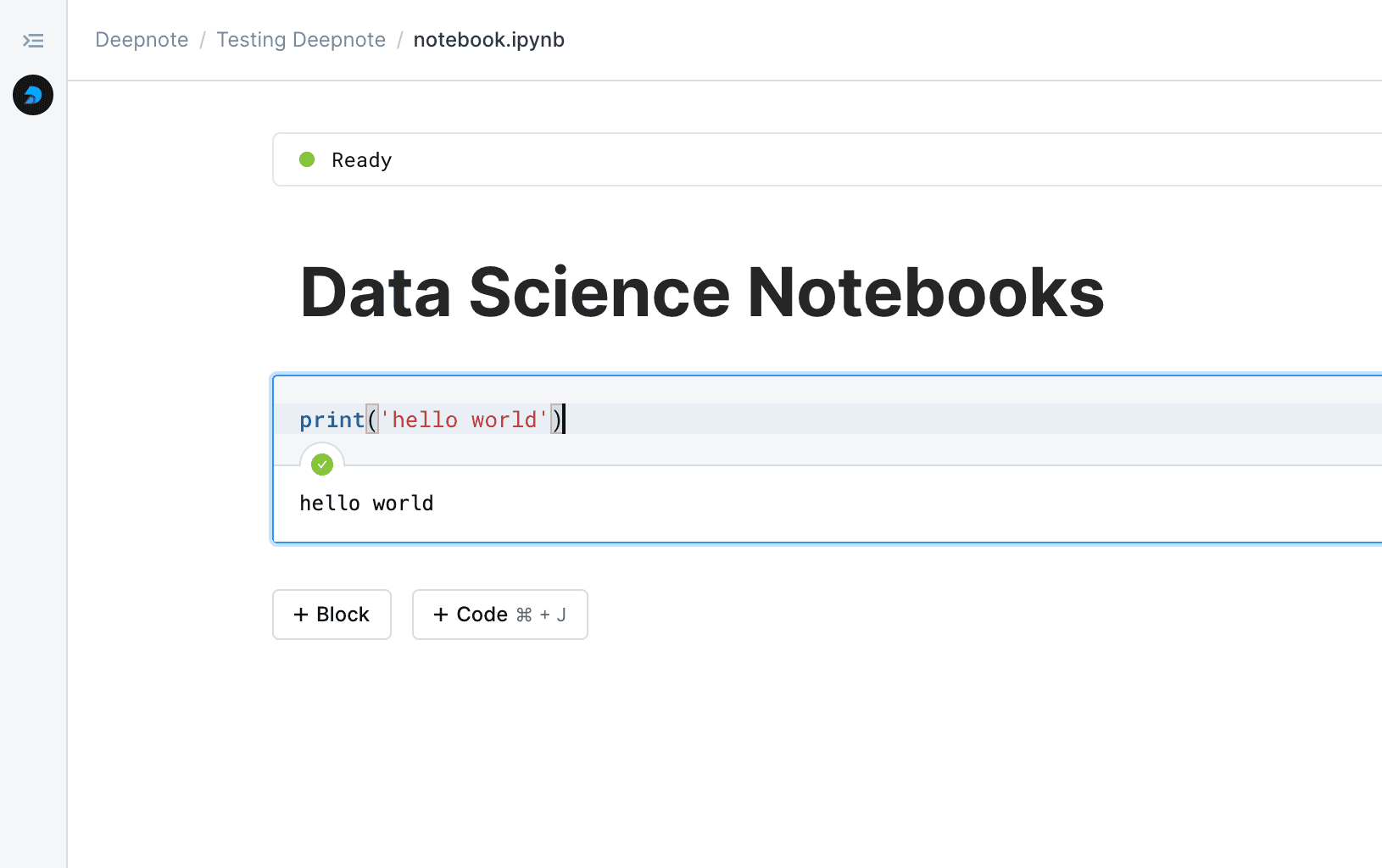
Deepnote
Mode Notebooks vs Deepnote
In the evolving landscape of data analytics platforms, Mode and Deepnote represent different approaches to enabling data work. While both platforms aim to facilitate data analysis and collaboration, they serve distinct needs within the analytics ecosystem.
Platform philosophy
Mode positions itself as a complete analytics platform, combining SQL-first analysis with Python notebooks and visualization capabilities. The platform emphasizes business intelligence and reporting alongside technical analytics, creating a bridge between data teams and business users.
Deepnote takes a more focused approach to data science collaboration, emphasizing notebook-based workflows while providing comprehensive integration capabilities and real-time collaboration features.
Core capabilities
Mode's approach combines multiple analytical paradigms. The platform integrates SQL exploration, Python analysis, and visualization tools into a cohesive workflow. This integration allows analysts to move seamlessly between SQL queries, Python analysis, and business reporting, making it particularly valuable for organizations bridging technical and business needs.
Deepnote provides a more traditional data science environment enhanced with modern collaboration features. The platform focuses on providing a comprehensive notebook experience with real-time collaboration and extensive integration capabilities, emphasizing flexibility and extensibility for data science teams.
Development environment
Mode's environment reflects its hybrid approach to analytics. The platform allows users to begin with SQL queries, transition to Python analysis, and create polished visualizations, all within a single workflow. This integration makes it particularly effective for teams that need to produce both technical analyses and business-ready reports.
Deepnote offers a more focused data science environment, emphasizing collaborative notebook-based workflows. The platform's interface balances accessibility with advanced features, supporting everything from exploratory analysis to production workflows while maintaining strong collaboration capabilities.
Data visualization and reporting
Mode places significant emphasis on visualization and reporting capabilities. The platform includes robust tools for creating interactive dashboards and shareable reports, making it particularly valuable for teams that need to communicate findings to business stakeholders.
Deepnote approaches visualization through a more technical lens, providing flexibility in creating visualizations through code while supporting various visualization libraries. The platform emphasizes the development process rather than final presentation, though it maintains strong sharing capabilities.
Collaboration features
Mode's collaboration features focus on sharing analyses and reports across technical and non-technical teams. The platform supports different levels of access and interaction, allowing organizations to maintain control over data access while facilitating broad consumption of analytics insights.
Deepnote emphasizes real-time collaboration among data professionals, with features designed for concurrent work on complex analyses. The platform enables simultaneous editing, commenting, and version control, creating an environment optimized for technical team collaboration.
Target audience
Mode serves organizations needing to bridge the gap between technical analysis and business consumption. The platform particularly suits teams that:
- Combine SQL and Python analysis
- Need to produce polished business reports
- Require robust visualization capabilities
- Work across technical and business teams
Deepnote caters to data science teams requiring a focused development environment. The platform excels for organizations that:
- Need comprehensive notebook functionality
- Value real-time collaboration
- Require extensive integration capabilities
- Focus on technical development
Use cases
Mode excels in scenarios requiring end-to-end analytics workflows, from initial data exploration to final business presentation. The platform's strength lies in its ability to serve both technical analysts and business users, making it particularly valuable for organizations needing to democratize data insights.
Deepnote shines in more technical scenarios, particularly those requiring sophisticated data science workflows and real-time collaboration. The platform's focus on development and integration makes it especially suitable for teams building complex analytical solutions.
Making the choice
The decision between Mode and Deepnote often reflects organizational needs and team composition. Teams requiring a balance between technical analysis and business reporting might find Mode's approach more aligned with their needs. Its combination of SQL, Python, and visualization tools creates an environment where different types of users can work effectively with data.
Organizations focusing on comprehensive data science workflows might find Deepnote more suitable. Its support for multiple programming languages and advanced features creates an environment well-suited to professional data science teams.
Future perspectives
Both platforms continue to evolve, with Mode expanding its capabilities while maintaining its focus on bridging technical and business needs. Deepnote continues to enhance its collaborative features and integration capabilities to serve modern data science teams.
Conclusion
Mode and Deepnote serve different needs in the data platform ecosystem. Mode excels in providing a comprehensive analytics platform that bridges technical and business users, particularly valuable for organizations seeking to combine SQL analysis, Python development, and business reporting.
Deepnote offers a more focused platform for data science teams, emphasizing real-time collaboration and development capabilities. Its support for multiple languages and extensive integration options makes it particularly valuable for teams requiring sophisticated analytical capabilities.
The choice between these platforms should align with your organization's specific needs and the balance you need to strike between technical development and business reporting. Understanding these distinctions helps ensure you select the platform that best supports your specific use cases while providing the necessary tools for team success.