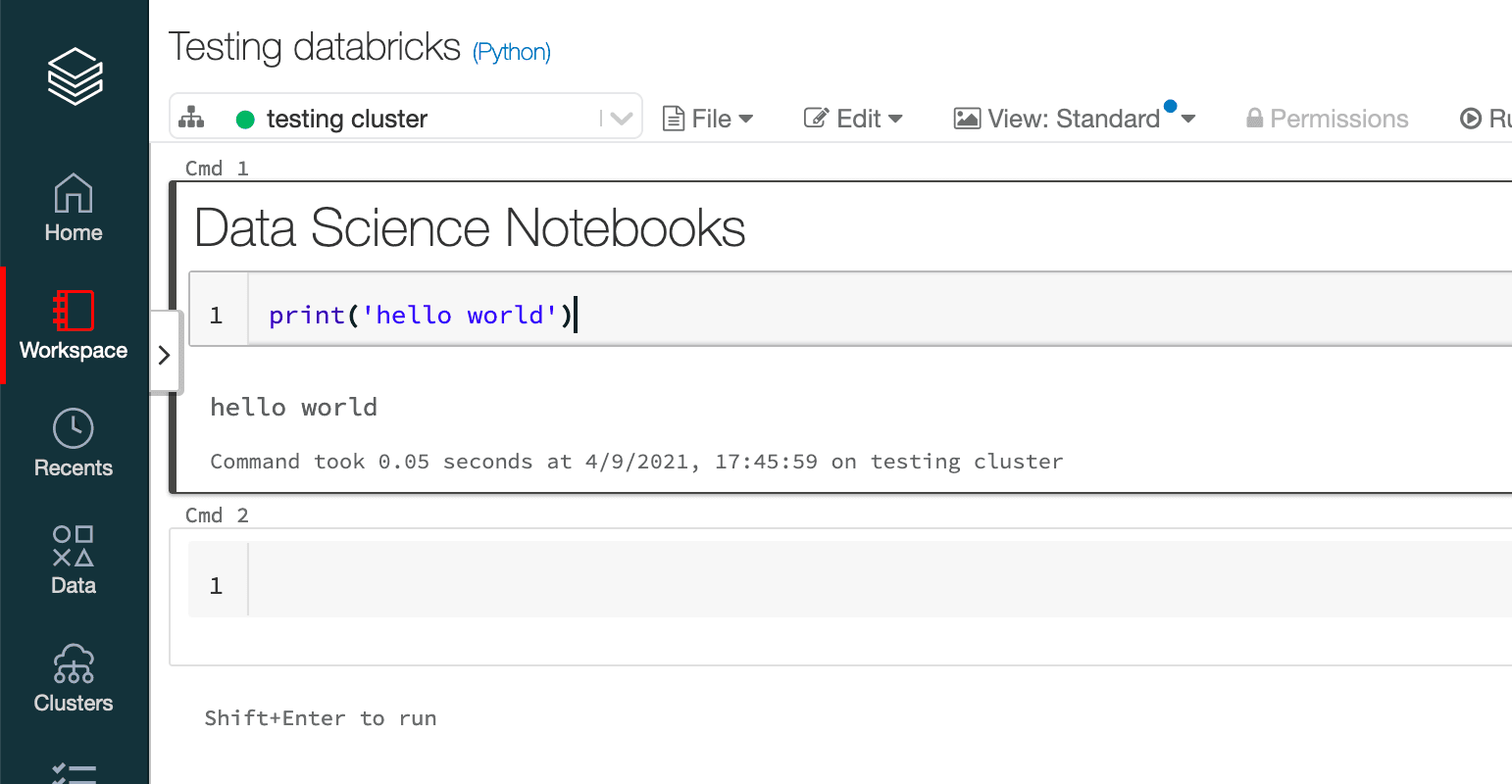
Databricks Notebooks vs Deepnote:
a side-by-side comparison for 2025
Comparing two data science notebooks.
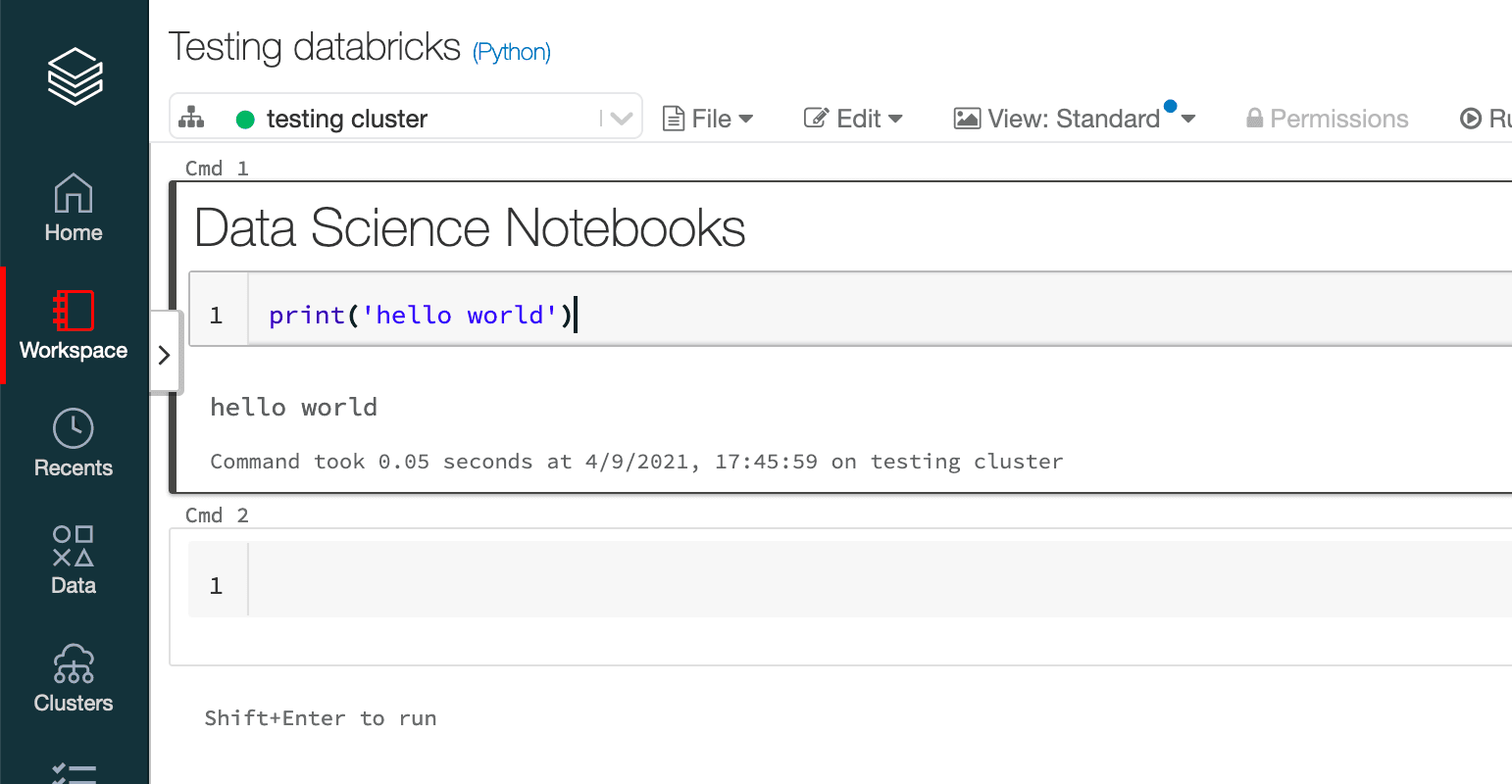
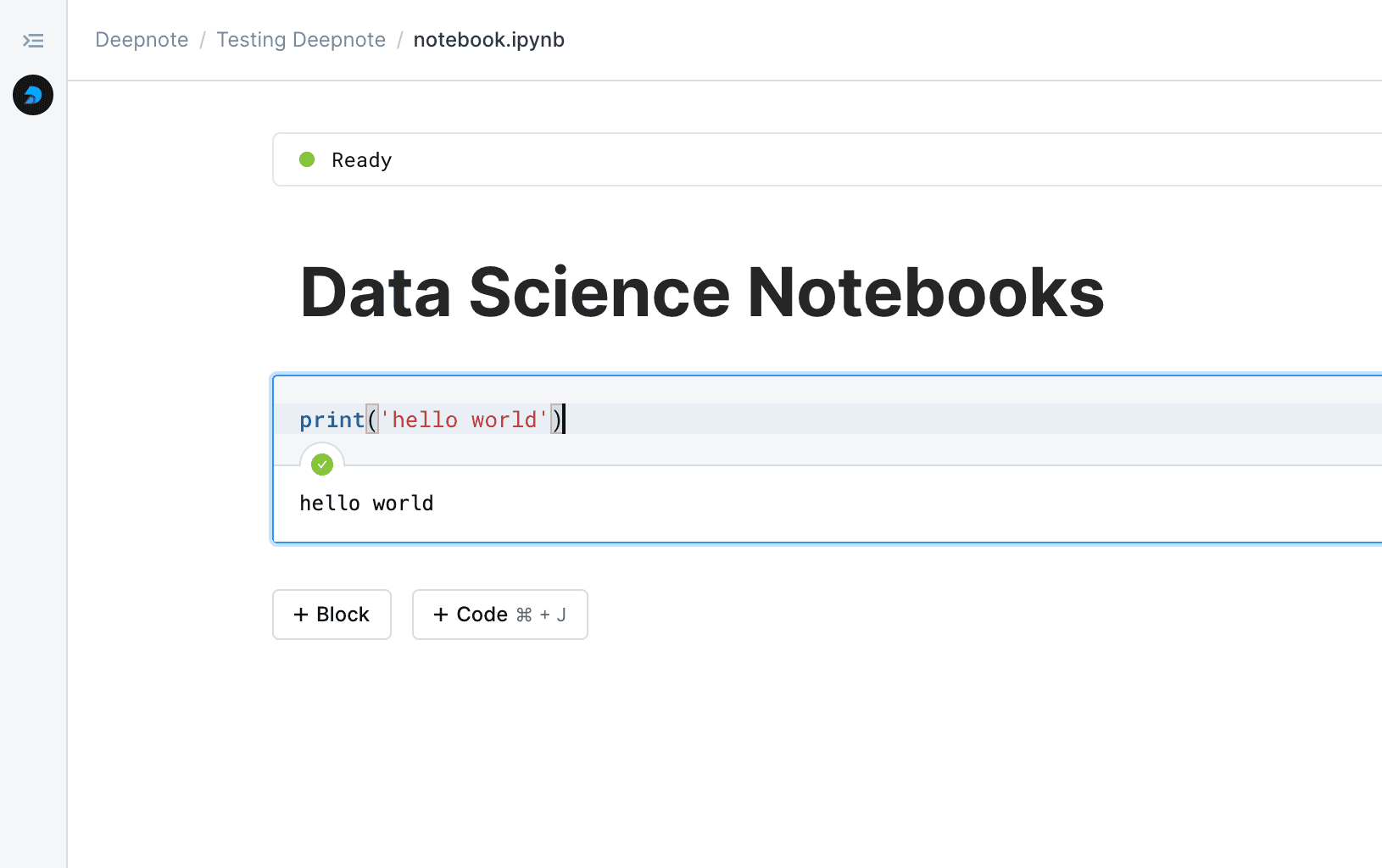
Deepnote
Databricks Notebooks vs Deepnote
While both Databricks and Deepnote operate in the data science space, they address fundamentally different challenges in the data ecosystem. Let's explore how these platforms compare and what makes each unique in their approach.
Databricks is an enterprise data platform built on Apache Spark, designed to handle massive-scale data processing and analytics. It excels at processing petabyte-scale datasets and managing complex data pipelines, with capabilities like materialized views that can efficiently handle 200+ billion row datasets. The notebook environment is just one component of its broader data lakehouse architecture.
Deepnote is a modern, collaboration-first data science platform that focuses on team productivity and accessibility. It provides an integrated environment for data exploration, analysis, and visualization, emphasizing immediate usability and team collaboration.
Key differences
Databricks is engineered for enterprise-scale data processing and analytics, capable of handling massive datasets with features like Delta Lake for data reliability and materialized views for performance optimization. Its notebook environment is part of a larger ecosystem designed for data engineering, warehousing, and ML operations at scale.
Deepnote, in contrast, approaches data science from a collaboration and productivity perspective. While it can handle substantial datasets, its strength lies in providing an integrated workspace for team-based data analysis and visualization. The platform emphasizes immediate usability, real-time collaboration, and AI-assisted development, making it ideal for data science teams focused on analysis and insight generation rather than massive-scale data processing.
Data processing and scale
At its core, Databricks leverages Apache Spark's distributed computing capabilities to handle petabyte-scale datasets efficiently. Its architecture is optimized for the data lakehouse paradigm, enabling organizations to process and analyze massive amounts of data with features like materialized views and Delta Lake integration. This makes it particularly powerful for enterprises dealing with big data challenges, such as processing hundreds of billions of rows while maintaining quick query response times.
Deepnote takes a different approach, focusing on data workflows and team collaboration. While it efficiently handles typical data science workloads, its strength lies in providing an intuitive environment for analysis and visualization. The platform offers seamless integration with various data sources and optimizes for interactive analysis rather than massive-scale processing.
Development environment
Databricks provides a Spark-native notebook environment with robust support for Scala and Spark SQL. Its integration with MLflow facilitates the complete machine learning lifecycle, making it suitable for production ML pipelines. However, this power comes with complexity - setting up and managing a Databricks environment requires significant expertise and resources.
Deepnote offers a modern, cloud-native notebook environment that prioritizes accessibility and collaboration. Its AI-assisted development features, including an autonomous agent for code generation and explanation, make it particularly appealing for data scientists and analysts. The platform's instant setup and intuitive interface reduce the barriers to entry, allowing teams to focus on analysis rather than infrastructure management.
Use cases and target users
Databricks shines in enterprise scenarios requiring large-scale data processing, such as implementing data warehousing solutions, running complex ETL operations, and deploying production ML pipelines. Its ability to handle real-time data processing and maintain a data lakehouse architecture makes it invaluable for organizations dealing with massive datasets.
Deepnote excels in scenarios focused on data science and analysis, where team collaboration and rapid iteration are crucial. It's particularly well-suited for exploratory data analysis, interactive reporting, and prototype development. The platform's emphasis on collaboration and accessibility makes it ideal for data science teams that need to work together effectively while maintaining high productivity.
Cost considerations
Databricks follows an enterprise pricing model based on compute and storage usage. While this model can be optimized for large-scale operations, it requires significant investment and careful resource management. The cost structure reflects its position as an enterprise-grade solution for big data challenges.
Deepnote offers a more transparent, team-based pricing model with a generous free tier. Its predictable costs and scalable pricing make it accessible to teams of various sizes, from small startups to larger organizations. This approach aligns with its focus on team productivity and collaboration rather than industrial-scale data processing.
Making the choice
Databricks is the clear choice for enterprises needing to process petabyte-scale datasets, implement a data lakehouse architecture, or run production ML pipelines. Its powerful distributed computing capabilities and enterprise-grade features make it ideal for large-scale data operations.
Deepnote, however, is the better choice for teams prioritizing collaborative data science work, analysis, and visualization. Its modern interface, AI-assisted development, and focus on team productivity make it perfect for organizations that need to enable effective collaboration among data scientists and analysts.
Conclusion
Databricks and Deepnote serve different segments of the data ecosystem, each excelling in its domain. Databricks stands out as an enterprise platform for massive-scale data processing and analytics, while Deepnote shines as a collaborative platform for data science teams focused on analysis and insight generation.
Consider your team's needs, scale of operations, and primary objectives when making the decision. Both platforms excel in their respective domains, but they address fundamentally different challenges in the modern data landscape. For enterprise-scale data processing and lakehouse architecture, Databricks is the clear choice. For team-based data science and analysis workflows, Deepnote offers a more suitable and accessible solution.