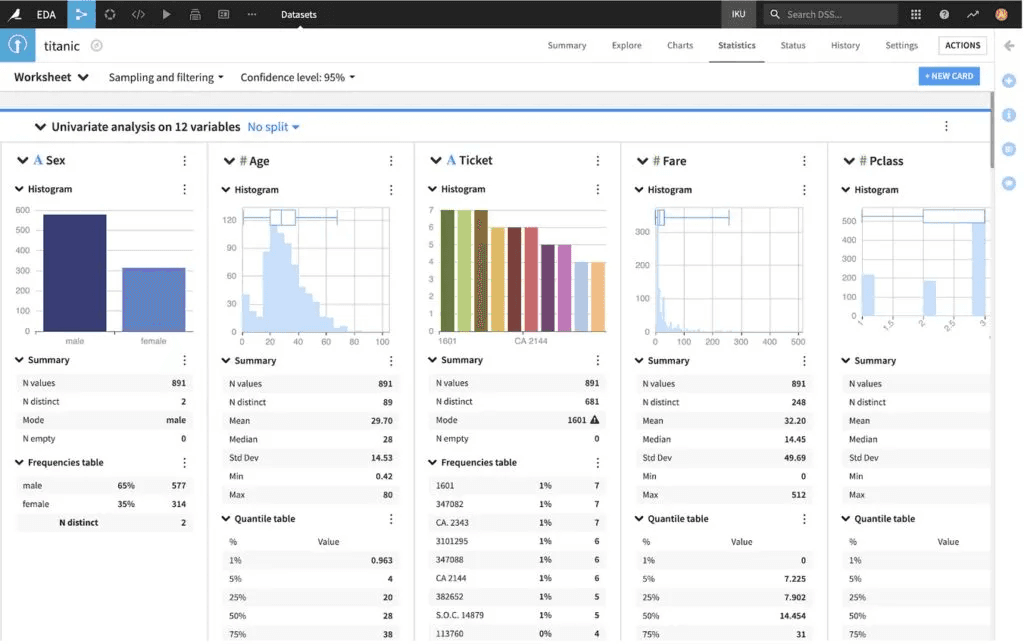
Dataiku vs Deepnote:
a side-by-side comparison for 2025
Comparing two data science notebooks.
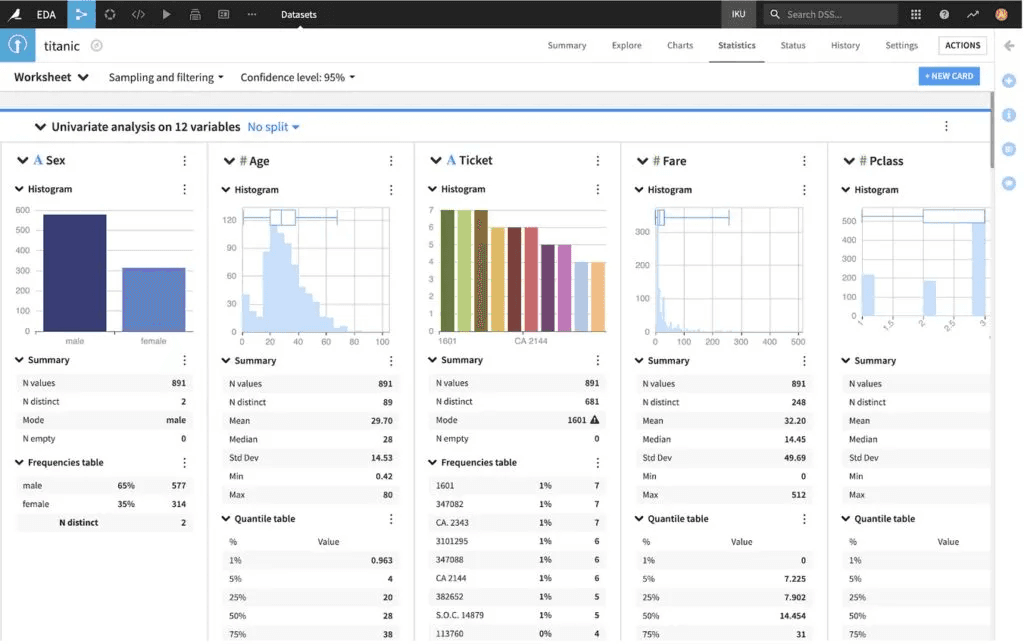
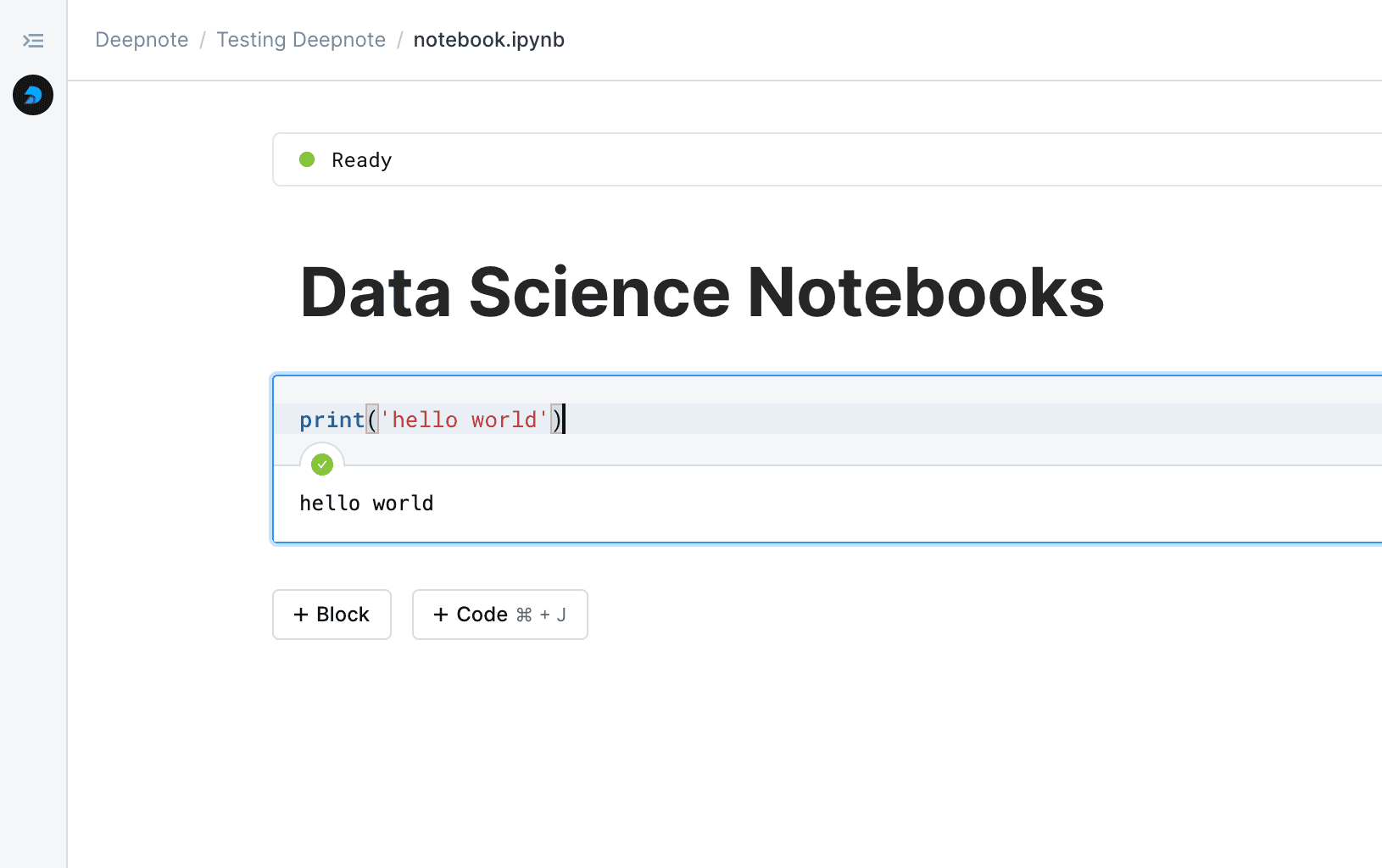
Deepnote
Dataiku vs Deepnote
In the evolving landscape of data science platforms, Dataiku and Deepnote represent two distinct approaches to empowering data teams. While both platforms aim to facilitate data science workflows, they cater to different organizational needs and working styles.
Platform philosophy
Deepnote embraces a modern, collaborative-first approach with its notebook-centric environment. The platform shines in its real-time collaboration capabilities, featuring an intuitive interface that seamlessly integrates AI assistance for both coding and visualization tasks. Its architecture supports immediate team productivity with built-in version control and multi-user editing capabilities.
Dataiku, on the other hand, positions itself as a comprehensive enterprise solution, offering an end-to-end platform for data science workflows. The platform's latest release introduces advanced features like multimodal machine learning and an enhanced LLM Mesh for AI integration, demonstrating its commitment to enterprise-scale innovation.
Technology and integration
Deepnote excels in modern stack integration, providing seamless connectivity with contemporary data tools and cloud services. Its notebook environment supports multiple programming languages while maintaining a clean, accessible interface that appeals to both beginners and experienced data scientists.
Dataiku emphasizes robust data preparation and AutoML capabilities, recently expanding its feature set to include advanced generative AI capabilities and enhanced governance tools. The platform's enterprise focus is evident in its comprehensive security features and scalable architecture designed for organization-wide deployment.
Market positioning and pricing
The platforms' pricing structures reflect their target markets. Deepnote maintains a flexible freemium model that appeals to growing teams and organizations, allowing them to scale their usage as needed. This approach makes it particularly attractive for startups and mid-sized companies looking for immediate value.
Dataiku targets enterprise clients with customized pricing plans, focusing on comprehensive organizational deployment and advanced features like multimodal machine learning and universal operations updates. Their latest release in 2024 reinforces this enterprise-centric approach with enhanced governance and collaboration features.
The decision between Deepnote and Dataiku ultimately depends on your organization's specific needs, scale, and data maturity level.
Choose Deepnote if:
- Your team values immediate productivity and real-time collaboration
- You need a modern, intuitive interface that reduces onboarding time
- You're looking for a flexible platform that can grow with your team
- Your workflow centers around interactive notebooks and quick iterations
- Budget consciousness and transparent pricing are important factors
Choose Dataiku if:
- You require enterprise-grade security and governance
- Your organization needs comprehensive AutoML capabilities
- You're looking for extensive data preparation and visualization tools
- You need robust project management features for large teams
- Your focus is on productionizing models at scale
Conclusion
While both platforms excel in data science, they target different needs. Deepnote stands out as an agile, modern solution with real-time collaboration and AI-assisted features, making it ideal for teams focused on quick iteration and immediate productivity. Its intuitive interface requires minimal setup, perfect for growing organizations.
Dataiku, conversely, offers a comprehensive enterprise solution with robust governance and AutoML capabilities. It's designed for large organizations needing extensive data preparation tools and enterprise-grade security.
Choose based on your scale: Deepnote for growing teams seeking agility and modern collaboration, Dataiku for enterprise-level operations requiring comprehensive governance and scalability.