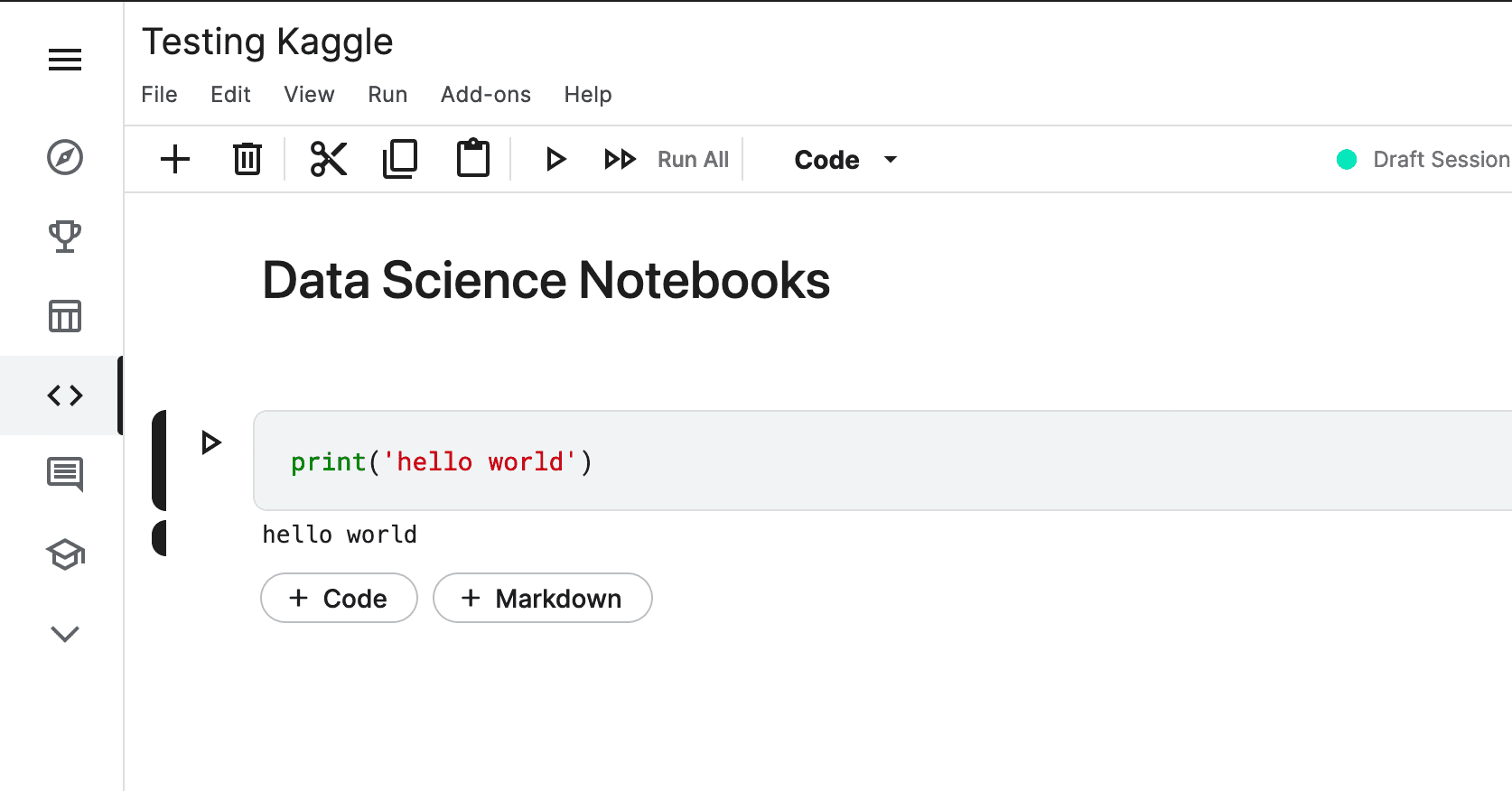
Kaggle vs Deepnote:
a side-by-side comparison for 2025
Comparing two data science notebooks.
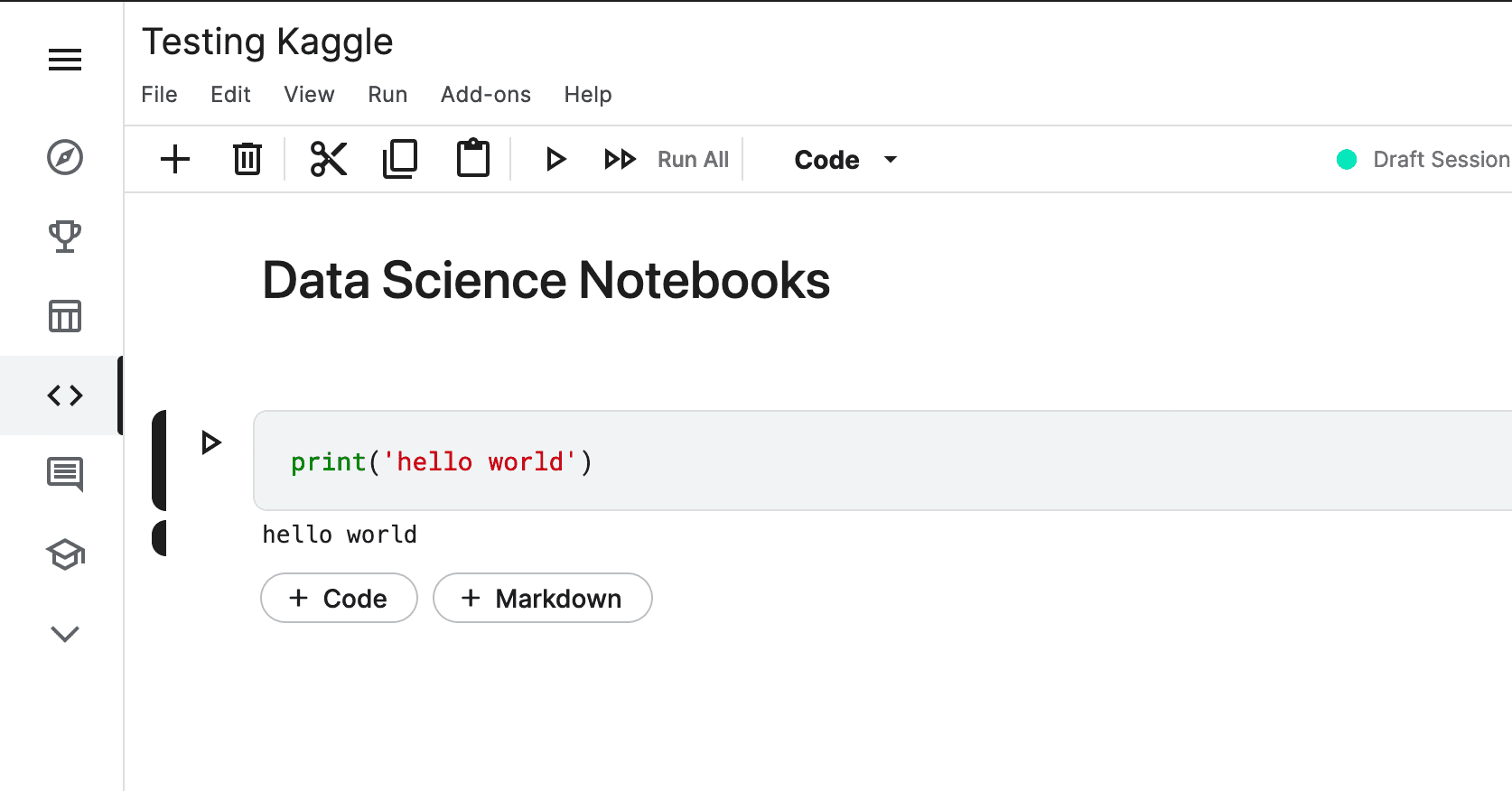
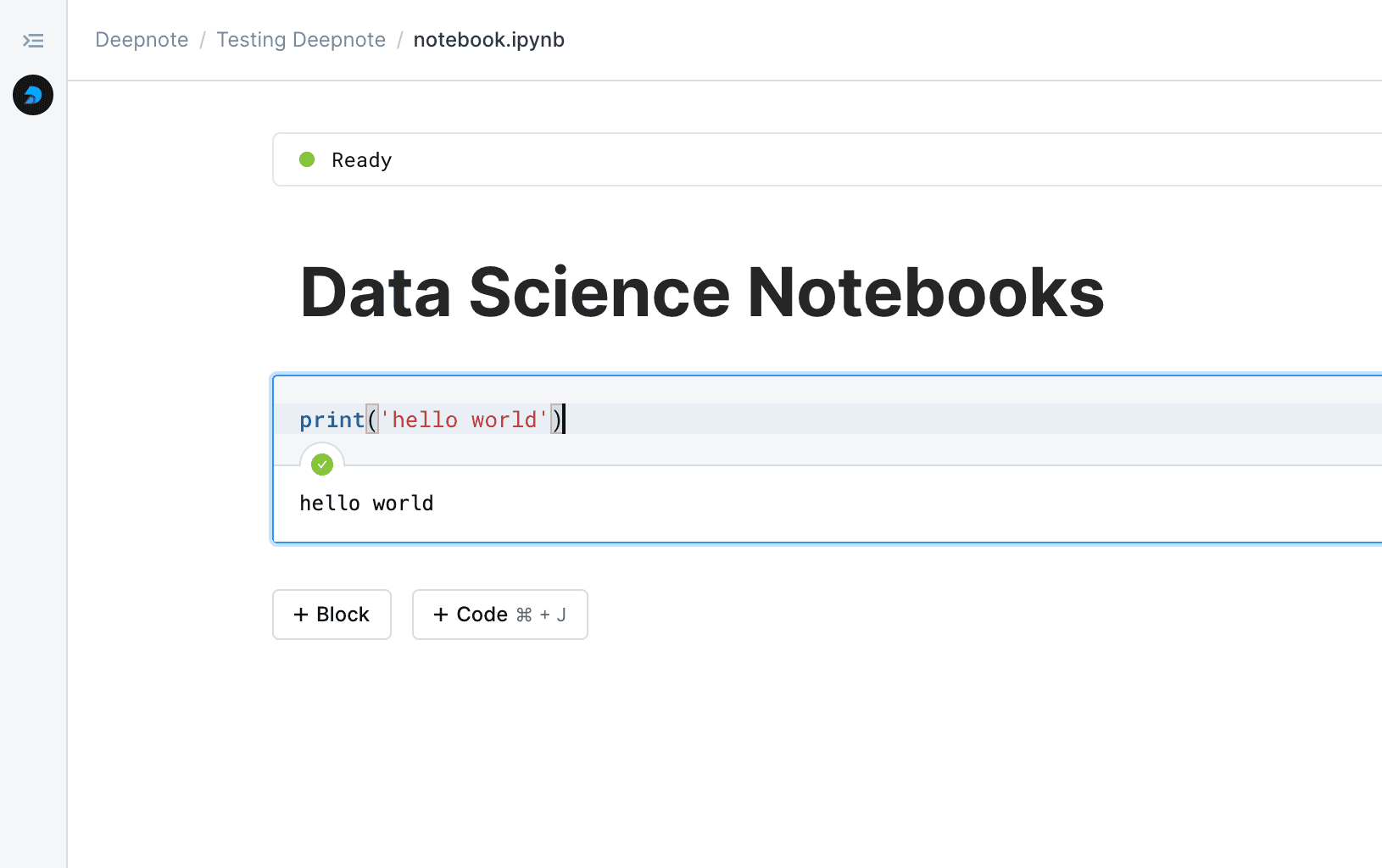
Deepnote
Kaggle vs Deepnote
In today's data-driven world, selecting the right platform for your data science work is crucial. Kaggle and Deepnote represent two distinct approaches to data science platforms, each with its unique strengths. While Kaggle has built its reputation on competitive machine learning and community learning, Deepnote positions itself as a modern, collaborative data workspace. Let's dive deep into what makes each platform unique.
Kaggle, owned by Google, is widely known as the world's largest data science competition platform and community. It provides a space for data scientists to compete, learn, and access vast amounts of public datasets. The platform has become synonymous with machine learning competitions and knowledge sharing, fostering a vibrant community of data enthusiasts and professionals alike.
Deepnote, on the other hand, is designed as a AI-powered data workspace that goes beyond traditional notebook functionality. It focuses on professional team collaboration, real-time editing, and seamless integration with a modern data stack. The platform emphasizes productivity and enterprise-grade features while maintaining an intuitive user experience that appeals to both individuals and teams.
Platform focus and core strengths
Kaggle's primary strength lies in its competition-based learning approach. The platform has revolutionized how data scientists learn and improve their skills through practical challenges. Its vast repository of public datasets and community-shared kernels creates an invaluable resource for anyone looking to enhance their data science capabilities. The competitive aspect drives innovation and encourages participants to push the boundaries of what's possible with machine learning.
Deepnote takes a different approach by focusing on professional workflow optimization. The platform has built a comprehensive environment where teams can collaborate in real-time, share insights, and develop sophisticated data solutions. Its modern interface and intuitive design make it accessible to both beginners and experienced professionals, while its advanced features cater to enterprise-level requirements.
AI and machine learning capabilities
When it comes to AI and machine learning capabilities, both platforms offer distinct advantages. Kaggle provides access to powerful computing resources through GPU and TPU allocation, essential for competing in machine learning challenges. The platform's competition-oriented structure naturally encourages users to experiment with cutting-edge algorithms and techniques.
Deepnote distinguishes itself through its integrated AI-assisted development features. The platform includes intelligent code completion, natural language querying, and automated data cleaning tools. These features significantly streamline the development process and help teams work more efficiently. The AI-powered visualization recommendations and code suggestions make it easier for teams to create professional-grade analyses and presentations.
Data connectivity and management
Data management approaches differ significantly between the two platforms. Kaggle's strength lies in its extensive public dataset repository, which has become a go-to resource for data scientists worldwide. The platform's focus on competition datasets has created a unique ecosystem where users can access high-quality, real-world data for learning and experimentation.
Deepnote takes a more comprehensive approach to data connectivity. The platform offers native integrations with major database providers and data warehouses, making it ideal for organizations working with diverse data sources. Its built-in data source management and visual exploration tools simplify the process of working with complex data structures and large-scale datasets.
Collaboration and team features
The collaboration models of these platforms reflect their different target audiences. Kaggle's collaboration features center around its community aspects, with forums, discussion boards, and team formation for competitions. This structure works well for knowledge sharing and community learning but may not suit all professional team needs.
Deepnote excels in professional collaboration scenarios. Its real-time multi-user editing capabilities, sophisticated version control, and integrated chat system create a seamless environment for team collaboration. The platform's permission management and workspace organization features make it particularly suitable for enterprise teams working on complex projects.
Learning and professional development
Kaggle's learning approach is deeply rooted in practical application through competitions. Users learn by participating in challenges, studying winning solutions, and engaging with the community. This competitive environment has proven extremely effective for skill development and keeping up with the latest trends in data science.
Deepnote facilitates learning through professional practice and team collaboration. The platform's focus on real-world applications and integration with modern development tools creates an environment where teams can learn while working on actual projects. The ability to create custom environments and share knowledge within teams makes it particularly valuable for professional development.
Making the choice
The decision between Kaggle and Deepnote should ultimately align with your specific needs and goals. Kaggle is an excellent choice for those looking to build skills through competition, access public datasets, and engage with a vibrant community of data scientists. Its competition-based structure provides unique opportunities for learning and skill demonstration.
Deepnote is the superior choice for professional teams requiring a comprehensive data science workspace. Its real-time collaboration features, advanced integrations, and focus on productivity make it ideal for organizations looking to streamline their data science workflows. The platform's modern approach to development and emphasis on team collaboration sets it apart in the professional space.
Conclusion
While both Kaggle and Deepnote serve the data science community, they excel in different areas. Kaggle's strength in competitions and community learning makes it invaluable for skill development and networking. Deepnote's focus on professional collaboration and integrated workflows makes it the go-to choice for team-based projects and enterprise solutions.
Consider using both platforms complementarily - Kaggle for learning and community engagement, and Deepnote for professional projects and team collaboration. The key is understanding your primary needs and choosing the platform that best aligns with your goals, whether they're focused on learning, competition, or professional development.
As the field of data science continues to evolve, both platforms will undoubtedly adapt and improve their offerings. What remains constant is their commitment to serving the data science community, albeit in different ways. Whether you're just starting your data science journey or leading a team of professionals, both Kaggle and Deepnote offer valuable tools and resources to support your success.