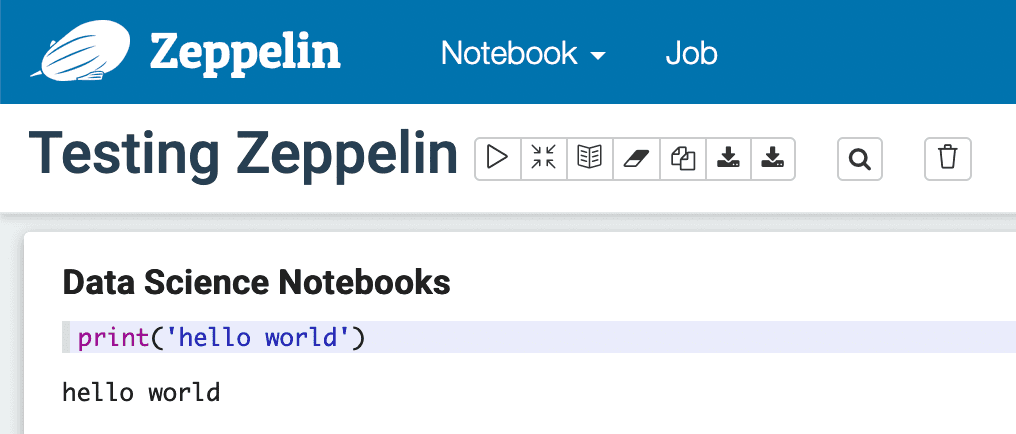
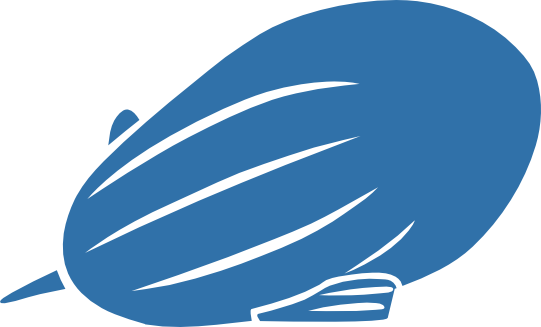
Comparing two data science notebooks.
Compare Zeppelin and Deepnote with other data science notebook tools.
Tool | Setup | Jupyter compatibility | Languages | Data visualization | Collaborative editing | Pricing | License |
---|
Zeppelin | Self-hosted | None | Python, SQL, Spark | Unknown | File-based or asynchronous collaboration | Free | Open source |
---|---|---|---|---|---|---|---|
Deepnote | Fully managed | Jupyter-compatible | Jupyter, SQL | Visualize with code or UI | Realtime collaboration | Free and paid options | Proprietary |
Amazon Sagemaker | Fully managed | Jupyter-compatible | Jupyter | Visualize with code | Limited collaboration | Free and paid options | Proprietary |
Jupyter | Self-hosted | Jupyter-compatible | Jupyter | Visualize with code | File-based or asynchronous collaboration | Free | Open source |
Google Colab | Fully managed | Jupyter-compatible | Jupyter | Visualize with code | Limited collaboration | Free and paid options | Proprietary |
Hex | Fully managed | Jupyter-compatible | Jupyter, SQL | Visualize with code or UI | Realtime collaboration | Free and paid options | Proprietary |
Databricks Notebooks | Self-hosted or fully managed | Jupyter-compatible | Jupyter | Visualize with code or UI | Realtime collaboration | Free and paid options | Proprietary |
DataCamp Workspace | Fully managed | Jupyter-compatible | Jupyter, SQL | Visualize with code or UI | Realtime collaboration | Free and paid options | Proprietary |
JupyterLab | Self-hosted | Jupyter-compatible | Jupyter | Visualize with code | File-based or asynchronous collaboration | Free | Open source |
CoCalc | Self-hosted or fully managed | Jupyter-compatible | Jupyter | Visualize with code | Realtime collaboration | Free and paid options | Open source |
Hyperquery | Fully managed | None | Python, SQL | Visualize with code or UI | Realtime collaboration | Free and paid options | Proprietary |
Jetbrains Datalore | Fully managed | Jupyter-compatible | Jupyter | Visualize with code or UI | Realtime collaboration | Free and paid options | Proprietary |
Kaggle | Fully managed | Jupyter-compatible | Jupyter | Visualize with code | Limited collaboration | Free | Proprietary |
Nextjournal | Fully managed | Jupyter-compatible | Jupyter | Visualize with code | Realtime collaboration | Free and paid options | Proprietary |
Noteable | Self-hosted or fully managed | Jupyter-compatible | Jupyter, SQL | Visualize with code or UI | Realtime collaboration | Free and paid options | Proprietary |
nteract | Self-hosted | Jupyter-compatible | Jupyter | Visualize with code | File-based or asynchronous collaboration | Free | Open source |
Observable | Fully managed | None | JS | Visualize with code | Realtime collaboration | Free and paid options | Proprietary |
Query.me | Fully managed | None | SQL | Visualization UI builder | File-based or asynchronous collaboration | Free and paid options | Proprietary |
VS Code | Self-hosted | Jupyter-compatible | Jupyter | Visualize with code | File-based or asynchronous collaboration | Free | Open source |
Mode Notebooks | Fully managed | None | SQL, R, Python | Visualization UI builder | File-based or asynchronous collaboration | Unknown | Proprietary |
Querybook | Self-hosted | None | Python | Visualization UI builder | Realtime collaboration | Free | Open source |
Count | Fully managed | None | SQL | Visualization UI builder | Realtime collaboration | Free and paid options | Proprietary |
Husprey | Fully managed | None | SQL | Visualization UI builder | File-based or asynchronous collaboration | Free and paid options | Proprietary |
Pluto.jl | Self-hosted | None | Julia | Unknown | File-based or asynchronous collaboration | Free | Open source |
Polynote | Self-hosted | None | Scala, Python | Unknown | File-based or asynchronous collaboration | Free | Open source |
Zepl | Fully managed | Unknown | Python, R | Unknown | Realtime collaboration | Unknown | Proprietary |