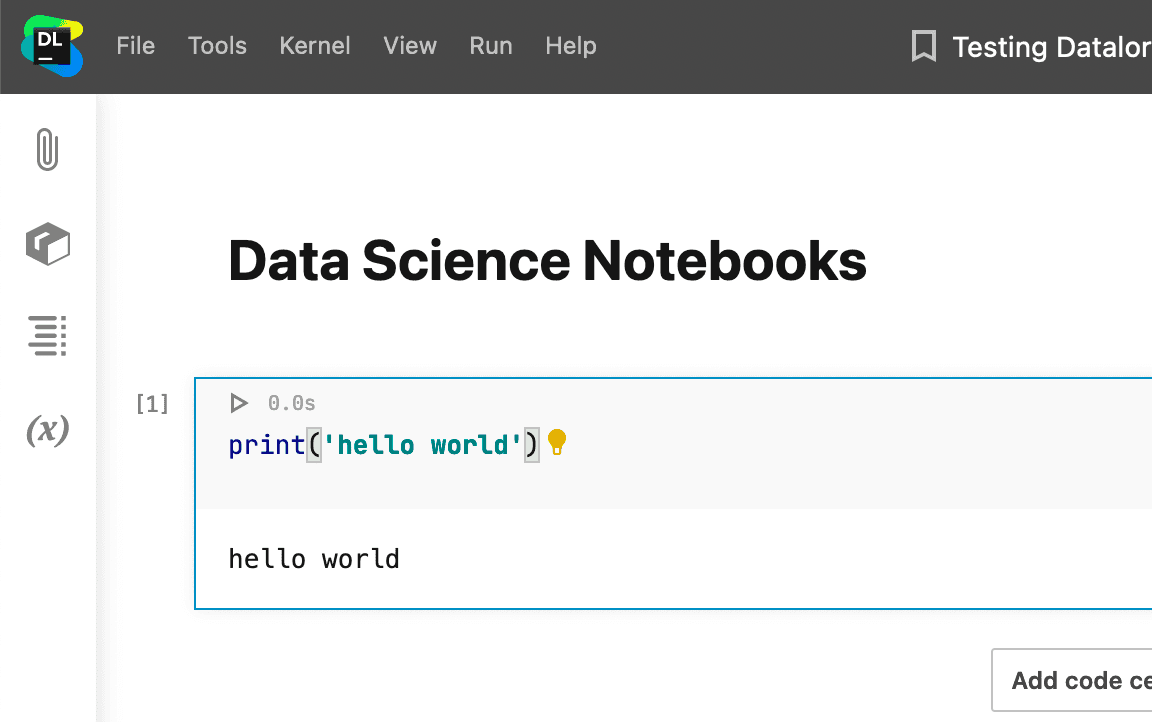
Jetbrains Datalore vs Deepnote:
a side-by-side comparison for 2025
Comparing two data science notebooks.
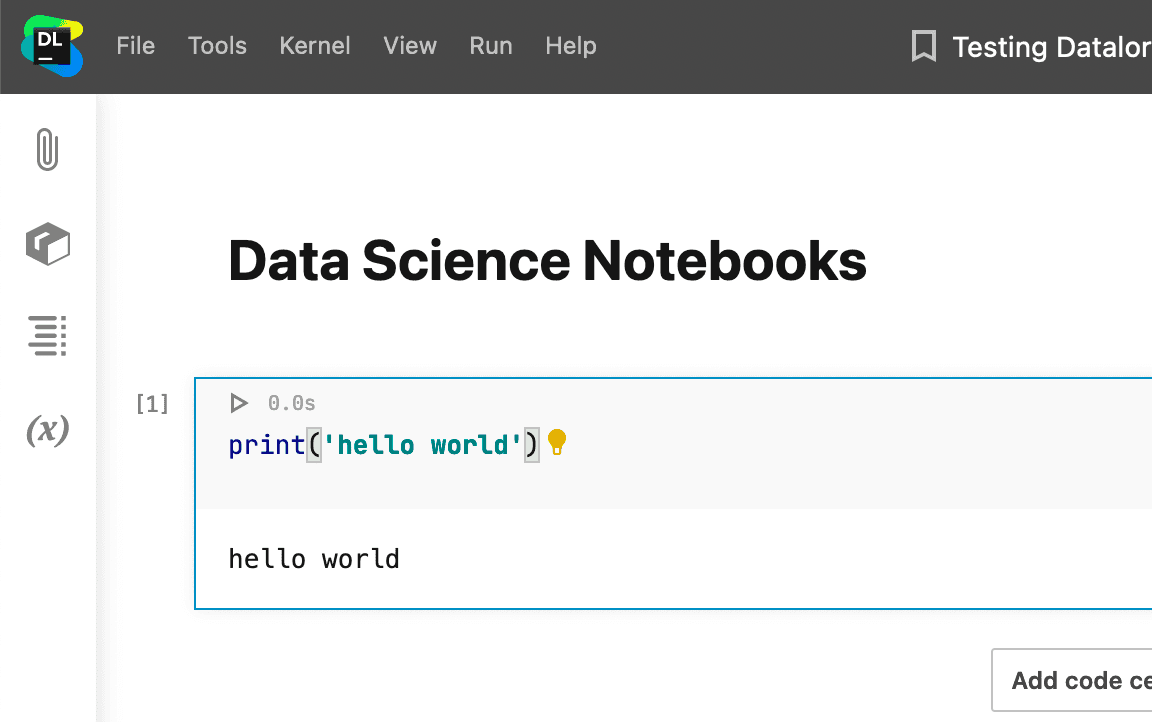
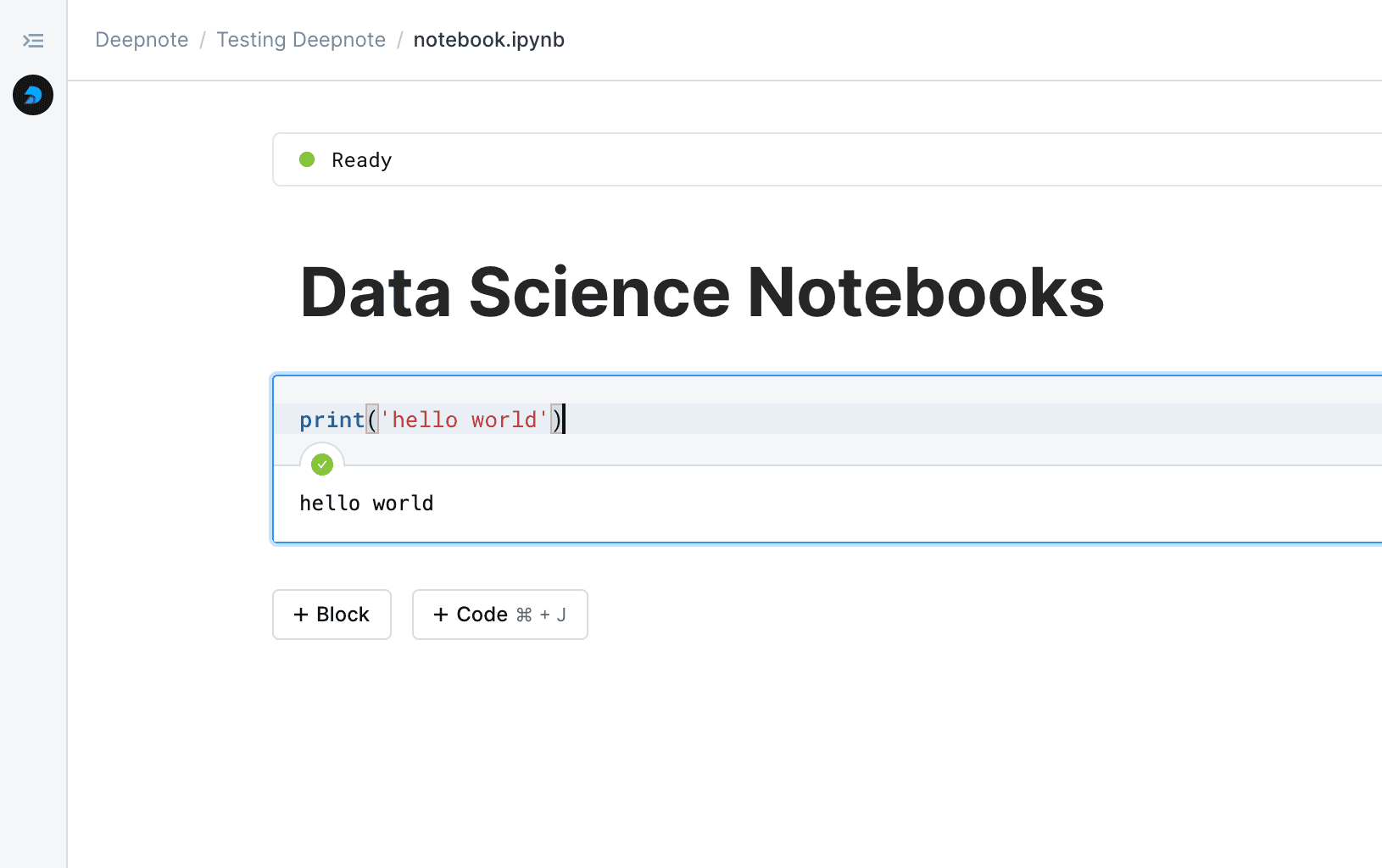
Deepnote
Jetbrains Datalore vs Deepnote
In this series of articles, we'll explore different data platforms and carefully assess their features. Our goal is to offer a fair and objective overview of the various data science solutions available. We will be examining Datalore and Deepnote.
Jetbrains’ Datalore is a collaborative data science platform. It is available in a managed cloud on the Community and Professional plans for personal use. The Datalore service can be hosted privately on the Enterprise plan or in a managed cloud with the Team plan (currently in Beta) for companies.
Deepnote is a collaboration first data platform. It provides a powerful, cloud-based workspace that allows users to easily explore, collaborate on, and share data, create interactive charts and dashboards, and build and deploy machine learning models.
Let’s break it down.
Jupyter Compatibility
Both Deepnote and Datalore platforms are entirely Jupyter compatible. You will not have to learn any new proprietary Notebook. You will encounter the same Jupyter you know and love. You can upload your Notebooks as an IPYNB and instantly begin working on your Notebook.
Exploratory Coding
Deepnote and Datalore both have built support for Python and R. The coding assistance comes from PyCharm for Datalore, whereas Deepnote uses state of the art AI code completion, provided by Codeium. Datalore also has built-in support for Kotlin and Scala.
Connecting to your data
Connecting to your various data sources, an essential part of data science, is handled eloquently by both platforms. Both Deepnote and Datalore feature a dizzying array of built-in data connectors for major cloud platforms, such as BigQuery, Snowflake, Redshift, Athena, and Clickhouse. This is complemented by both platforms with an easy drag-n-drop for CSVs. Deepnote has the slight edge in file based connections, where it has first class integrations with Google Drive, Google Cloud Storage, Amazon S3, Dropbox, OneDrive, and Google Sheets. Deepnote also allows for first class integrations with version control software such as Git and GitLab.
Interacting and visualizing your data
SQL is an important chunk of any notebook, and both Deepnote and Datalore have you covered with Notebook SQL blocks, which come equipped with code completion and built in data frame outputs. Both apps come with built-in charting solutions, while still allowing users to use all python charting libraries with ease.
Publishing your data
If you don’t report your findings, did you find anything at all? Both Deepnote and Datalore have fully fledged reporting and app tools. Both platforms make it easy to schedule your notebook, prepare dashboards, and even have interactive dashboarding. Both with fine grade permissioning that allows organizations to share reports publicly and with in the organization.
Collaboration
It comes as no surprise, that since both platforms bill themselves as collaboration first, that both platforms share a numerous amount of features meant to increase collaboration. Fine tuned permissions, collaborative notebooks, multi-user editing, commenting.
AI
Both platforms feature in block AI to help you write, fix, and explain your code. As of the time of writing however, Deepnote has a best-in-class AI autonomous AI agent that is able to create SQL blocks, Python Blocks, text blocks, all while running the notebook to make sure that it is getting the expected results, and fixing itself if it isn't.
Pricing
Deepnote comes equipped with a free tier, and a free two week trial on signup, with no credit card needed. Datalore also has a free tier, and also offers a two week free trial. Where they differ is that Datalore has a team and individual plan, the team plan is more expensive than Deepnote, and requires a minimum of three team members. Deepnote’s team plan has no minimum seat requirement. Of course with enterprise plans, both platforms want you to talk to their respective sales teams.
Conclusion
Both Datalore and Deepnote are collaborative-first data platforms with similar feature sets, designed to address common user needs. Your choice may depend on your specific requirements. If you value first-class support for Scala and Kotlin, Datalore is an excellent choice. For advanced AI integration and a wide range of integrations, Deepnote is a strong contender. Regardless of your decision, thank you for reading, and we hope you enjoy your data science journey.